- How Predictive Analytics Works
- What is Predictive Analytics in Machine Learning?
- Difference Between Data Analytics and Predictive Analytics
- How Do Big Data and Predictive Analytics Help Businesses?
- Data mining and predictive analytics are the same?
- Here are some Detective Techniques Used in Data Mining:
- Data Mining and Predictive Analytics Working Together for Success
- Why Do We Need Predictive Analytics?
- The Future of Predictive Analytics
Imagine knowing when your computer might crash before it happens! Predictive analytics in IT uses computer programs that analyze past system logs or sensor readings to predict potential problems before they arise.
How Predictive Analytics Works
- At its core, data analysis works like looking at your computer's activity monitor - giving an instantaneous account of what's taking place now like how much CPU your programs are utilizing.
- Predictive Analytics works like your activity monitor in terms of predicting when your computer might slow down. It analyzes past data such as CPU spikes before crashes to forecast future events.
Machine learning algorithms perform these predictions with great precision. Machine learning uses past data to learn, just like an experienced IT technician could identify warning signs before their system crashes; using this knowledge, machine learning predicts potential issues before they arise.
An IT department might use data analysis to assess how much memory your computer is currently utilizing; with predictive analytics, however, they can attempt to predict when you might run out of space based on your workload and historical usage patterns - taking preventative steps like freeing up space or upgrading RAM as necessary to avoid crashes or slowdowns.
Many organizations rely on predictive analytics in IT to boost system performance and avoid downtime, using data analysis to forecast potential problems before they occur. It can be an extremely powerful tool.
What is Predictive Analytics in Machine Learning?
Predictive analytics in machine learning uses algorithms that adapt and learn from data to make predictions. Unlike traditional statistical models, machine learning models can handle large volumes of data more efficiently while uncovering patterns not visible to human analysts. As these models gain experience they become better predictors. These predictive abilities also increase over time due to greater exposure.
Predictive analytics begins with data mining, in which companies examine different datasets and search for patterns within them. Once collected, this information is fed into machine learning algorithms which use it to detect trends or anomalies that could impact future results and detect outliers -- data points that differ significantly from their average values within any particular dataset.
Once the data has been processed and analyzed, businesses can segment customers based on certain characteristics to more effectively target marketing campaigns and product offerings.
For instance, using predictive analytics can identify customers who may be at risk of leaving and offer them discounts to keep them loyal. It can also reduce fraud by detecting suspicious activity such as credit card fraud or any type of illicit behavior through transaction history analysis that highlights patterns that suggest this kind of fraudulent behavior.
Banks use predictive analytics to assess customer risk profiles before extending loans. Likewise, every organization prioritizes data security; predictive analytics can pinpoint patterns associated with suspicious end-user behavior that trigger appropriate security procedures.
Difference Between Data Analytics and Predictive Analytics
Data analytics forms the core of predictive analytics. Data analysts gather, cleanse, and examine information to gain meaningful insights from it. However, predictive analytics takes this a step further by employing sophisticated statistical techniques, machine learning algorithms, and modeling approaches to uncover hidden patterns within historical data sets and use this insight for forecasting purposes in the future.
Consider it this way: data analytics allows us to comprehend what has happened and is happening now, while predictive analytics gives us the power to anticipate what may occur next. Machine learning plays a pivotal role in predictive analytics: algorithms trained on large datasets learn patterns and relationships within them that allow them to identify new patterns as well as make predictions about upcoming events.
An e-commerce website uses data analytics to understand customer demographics, purchase histories, and browsing behavior. However, using predictive analytics can go further in terms of product prediction: it allows the website to personalize product recommendations while improving customer satisfaction and ultimately increasing sales.
How Do Big Data and Predictive Analytics Help Businesses?
Think of big data as an immense mountain of information: customer clicks, sensor readings, and social media posts are just waiting to be analyzed. All this data provides fuel for predictive analytics which becomes smarter and more accurate as more is collected - the more data is available the greater its ability to learn and predict future trends.
Let's see how these two can be applied in practice:
- Enhanced Customer Experience: Predictive analytics helps businesses create happier customers through personalized experiences for customers. Imagine an online store offering products you may actually like rather than random suggestions; this would keep customers satisfied and keep coming back for more! This keeps businesses happy while simultaneously improving the bottom line.
- Improved Risk Management: Predictive analytics is like having Superman's sixth sense: it allows businesses to quickly detect any impending problems before they occur and act as a barrier against security threats or fraud attempts. By analyzing data patterns, IT teams can identify suspicious activities quickly and prevent costly issues before they arise.
- Optimized Operations: Predictive analytics allows us to predict when servers may crash or equipment needs maintenance by analyzing sensor data and historical trends, which enables proactive maintenance to avoid downtime that costs companies both money and productivity.
- Informed Decision-Making: Data tells its own tale; predictive analytics makes sense for businesses to make smart decisions regarding product development and resource allocation - leading to improved strategies and overall success.
Real-World Example:
Imagine a factory where many machines are continually in operation. By analyzing sensor data and past maintenance records, predictive analytics can predict when one may fail and enable preventive maintenance - saving cost in downtime while keeping production on schedule.
Data mining and predictive analytics are the same?
Both data mining and predictive analytics offer insight from existing datasets; their primary difference lies in how this occurs.
Data mining involves searching through large datasets for patterns. It primarily aims at discovering correlations and associations that may aid business decisions, as well as exploring relationships in time series data or other types of information such as photographs.
Examples of data mining techniques include association rules (a form of market basket analysis that detects items purchased together), or clustering which groups data points according to similarity.
Discoveries like these help businesses better understand their customers, which allows for targeted marketing campaigns, optimized product offerings, and forecast sales projections - leading to higher returns on investment for companies. In addition, fraud detection capabilities help reduce financial risk while increasing security, as well as fulfilling regulatory compliance obligations and making the most out of marketing budgets.
Here are some Detective Techniques Used in Data Mining:
- Sorting (Classification): This is like putting similar things together. Imagine sorting customers into "high spenders" or "frequent buyers" based on their purchase history. Banks might use this to decide if someone can get a loan.
- Grouping (Clustering): This is like finding groups with something in common. A music streaming service could use this to find groups of people who listen to similar music.
- Finding Connections (Association Rule Learning): This is like figuring out what things go together. A grocery store might use this to see what products people often buy together (like milk and cookies) to improve store layout.
Data Mining and Predictive Analytics Working Together for Success
Data mining is like the detective finding clues, and predictive analytics uses those clues to predict the future. By working together, they help businesses unlock the hidden potential within their data and make better decisions!
Why Do We Need Predictive Analytics?
Predictive analytics offers a multitude of benefits for businesses and organizations of all sizes. Here are some compelling reasons why you should consider incorporating predictive analytics into your strategy:
- Foresight is King: To stay ahead of competitors and meet future customer needs, anticipate future trends and needs in advance to enable proactive decision-making - like launching products or services that resonate with your audience.
- Reduce Costs: Pinpoint areas for improvement and optimize operations using predictive analytics to eliminate wasteful spending and ineffective practices, leading to considerable cost savings.
- Proactive Protection: Anticipate potential business disruptions by taking preventative steps before they arise - be they equipment failure, security breaches, or customer churn. By doing this, you'll minimize downtime while protecting assets.
- Data-Driven Decisions: Forget intuition-based decisions! Predictive analytics provides invaluable data-driven insights that can inform your strategic choices with evidence instead of mere intuition. Make strategic choices supported by evidence, not guesswork.
- Enhance Customer Relationships: Create lasting customer relationships by anticipating their needs and preferences, offering personalized offerings and recommendations to create an improved customer experience, and fostering loyalty.
Predictive analytics isn't about star gazing; rather, it involves using data to make smarter decisions, navigate uncertainty more successfully, and achieve long-term success.
The Future of Predictive Analytics
Predictive analytics offers many possibilities. As AI and machine learning advance, predictive models will be more accurate. We can expect to see advances in:
- Artificial Intelligence (AI): This field specializes in creating AI models that are more transparent and interpretable so that users can better understand how the algorithm reached their predictions.
- Real-Time Analysis: The ability to analyze data instantly and make predictions will become more important as businesses can react instantly to changing circumstances.
- Integrate IoT Devices: IoT devices can provide massive amounts of data, which will allow you to create predictive models that have great predictive power.
Predictive analytics is no longer science fiction. It has become a vital business tool for all industries. As businesses compete for survival and growth, it will be more important than ever to predict future outcomes using data.
Conclusion
Predictive Analytics is a new field that uses data and AI to forecast future events and trends. Understanding the difference between data analytics and prediction analytics, as well as big data's vital role and data mining's agent role in predictive modeling will help you unlock its full potential. You can use predictive analytics to make informed decisions that will lead to success today and in the future.
Web and mobile app developers rely on predictive analytics to increase user engagement and retention, by understanding user behaviors and preferences to personalize content, recommend products, optimize experiences, identify issues for improvement as well as increase performance resulting in happier app performance and greater satisfaction among their audience.
Predictive analytics provides software development companies with numerous advantages during the software development process. By forecasting project timelines, identifying bottlenecks, and forecasting resource needs accurately, companies can improve project management while still meeting timelines to deliver high-quality products on schedule. In addition, predictive analytics also aid in understanding market demands which enable software that addresses specific audience demands.
FREQUENTLY ASKED QUESTIONS (FAQs)
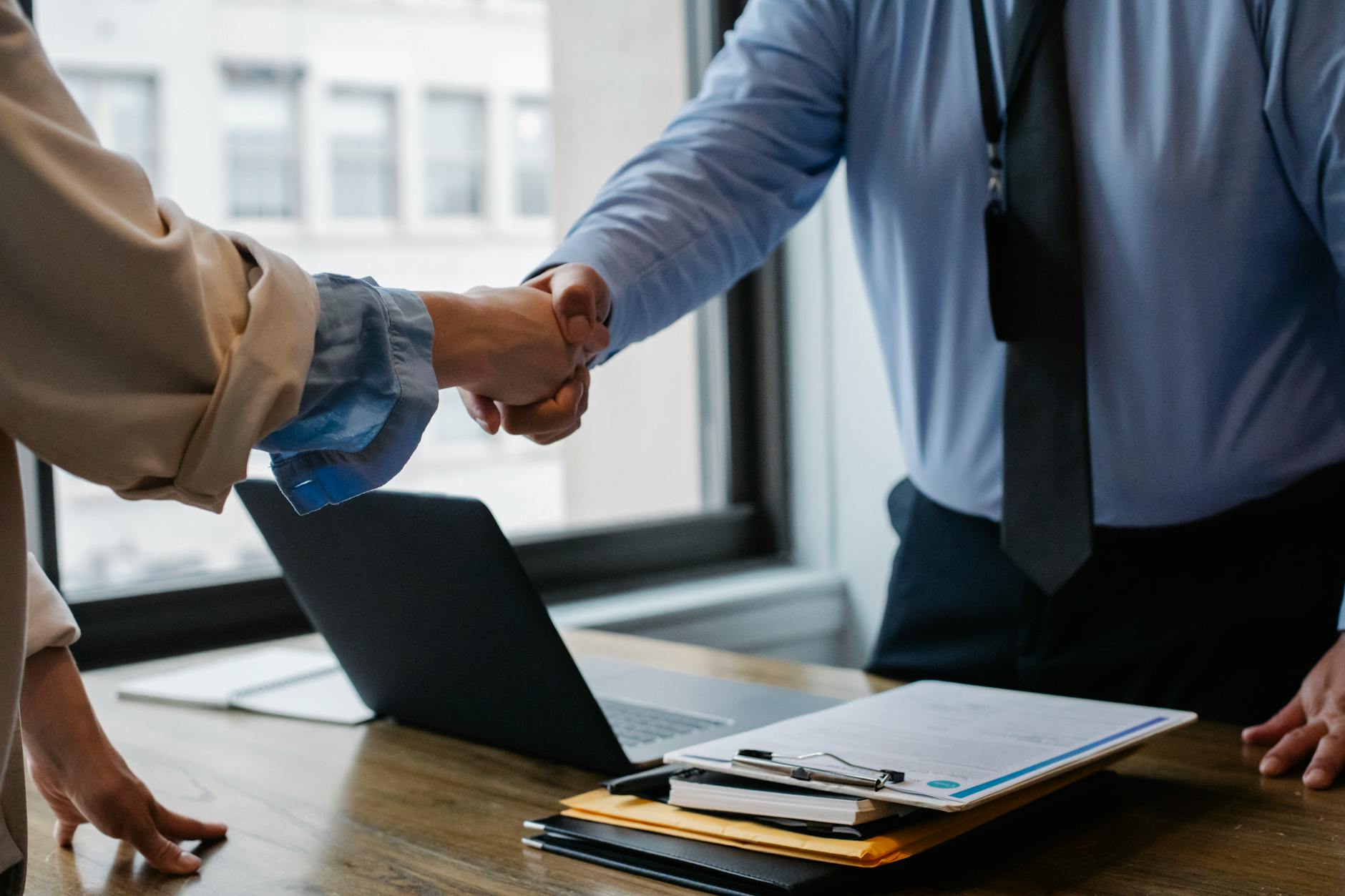